Simple Random Sampling
Last Updated :
13 Nov, 2023
It is frequently impractical or even impossible to gather data from the full population in the subject of statistics. Under such circumstances, researchers resort to sampling techniques, simple random sampling being one of the most basic. Using simple random sampling, a subset of people or things is chosen from a wider population, giving every person in the population an equal chance of being included. To conclude a population, this method is crucial as it offers a representative sample for statistical analysis.
What Is Simple Random Sampling?
Using simple random sampling, every person in the population has an equal and independent probability of being chosen for the sample. It is a fundamental component of statistical research since it is a completely random method that reduces bias and guarantees the representativeness of the sample.
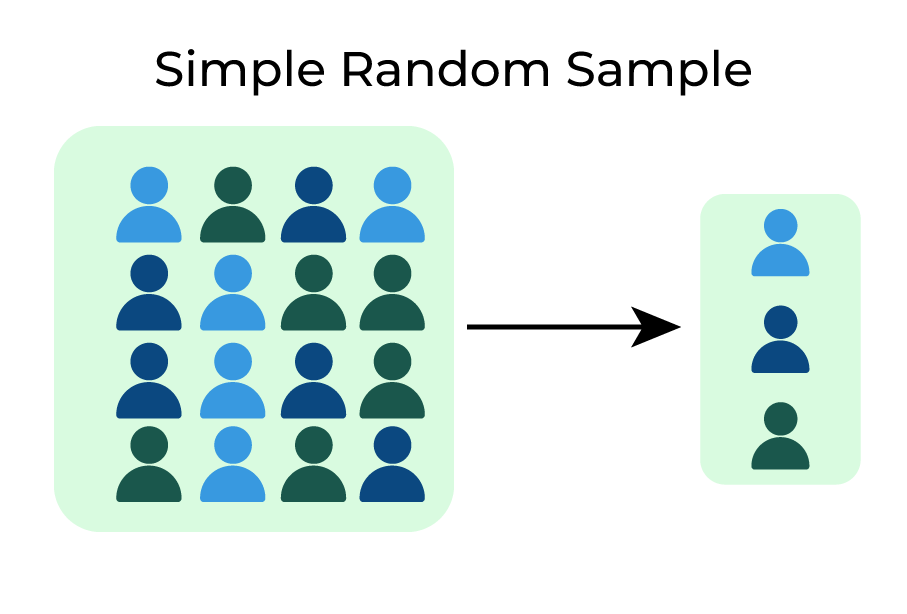
Assumptions for Simple Random Sampling
- Equal Probability: Every member of the population must have an equal chance of being chosen in a basic random sampling. That is, no element has a higher or lower chance of being selected than any other.
- Independence: The probability that other elements will be selected shouldn’t be impacted by the choice of one element for the sample. Every option stands alone from the ones that came before it.
- Randomness: There should be no discernible pattern or bias in the selection process; it should be random. This makes it more likely that the sample will accurately reflect the total population.
Key Concepts of Simple Random Sampling
Here find the Key Concepts of Simple Random Sampling:
- Population and Samples
- Population: It is the total set of people or things that you wish to research or draw conclusions about. Although studying every person of the population is either impracticable or too expensive, it reflects the bigger group that you are interested in.
- Sample : A portion of the population is called a sample. It is a smaller subset of the population that has been chosen with the goal of being representative of the whole. Your goal in analyzing the sample is to make inferences about the total population.
- Sampling Frame : A list or an ordered representation of every person or thing in the population is called a sampling frame. It acts as the foundation for choosing the sample. To prevent bias in the sample selection process, the sampling frame must be thorough and accurately represent the population.
- Randomization: An essential component of basic random sampling is randomization. It denotes that each person or thing in the population has an independent, equal chance of being chosen for the sample. By using this procedure, it is guaranteed that the sample is unbiased and accurately represents the population. Drawing lots, random number generators, and other equitable techniques can all be used to achieve randomization.
- Sample Error:The discrepancy between the features of the sample and the population it represents is known as sampling error. It happens as a result of researching merely a sample, or a subset, rather than the full population. Randomness in the sampling process naturally leads to sampling mistake.
For example, You wish to determine the average height of all high school students in a city . You determine the average height of 100 students by randomly selecting a sample, as instead of measuring every student, which is unfeasible. Given that the population’s average height may vary significantly, sampling error is probably present if the average height in your sample is 160 cm. By expanding the sample size, the magnitude of this inaccuracy can be minimized.
- Non-sampling Errors: These are mistakes that have nothing to do with the actual sample procedure. These can happen at different phases, such gathering, analyzing, or interpreting data.
- Calculating Sample Size for SRS: To attain a particular level of accuracy or confidence in your study, you must calculate the sample size for Simple Random Sampling (SRS) by figuring out how many people or items you should include in your sample. The population size, margin of error, and intended confidence level are among the variables that affect it.
For example ,let’s imagine you wish to poll the population of a city to find out how many people favor a specific policy. If your prediction is accurate to within three percentage points, you should have 95% confidence in it. Ten thousand people live in the city. You would utilize statistical formulas or online calculators that account for the population size, margin of error, and desired confidence level in order to determine the sample size. The outcome may indicate that a sample size of 385 respondents is required. This implies that in order to draw conclusions about the total population, you should poll and choose 385 residents at random.
- Bias: In simple random sampling (SRS), bias is the term used to describe a systematic error that may transpire throughout the sample selection process, rendering the sample unrepresentative of the population. Inaccurate or biased conclusions about the population might result from bias.
For example, consider that you wish to research the eating patterns of every university student. You choose to survey a random sample of students, but you only poll those who are accessible during lunch on a given day. Since your sample is primarily composed of students with flexible schedules, it will be biased if a large number of students have class during that period and are unable to participate. Inaccurate judgments about the entire student body may result from this bias.
Benefits of Simple random sampling
Here are some benefits of simple random sampling:
- Unbiased: Simple random sampling is an unbiased method of sampling, meaning that every member of the population has an equal chance of being selected into the sample. This is important because it ensures that the sample is representative of the population.
- Easy to implement: Simple random sampling is a relatively straightforward method to implement. All you need is a complete and accurate sampling frame and a random number generator.
- Flexible: Simple random sampling can be used to sample from a wide variety of populations. It can be used to sample from small populations, large populations, homogeneous populations, and heterogeneous populations.
- Generalizable: The results of a simple random sample can be generalized to the population as a whole. This means that you can use the results of your sample to make inferences about the population.
- Transparent: Simple random sampling is a transparent method of sampling. This means that it is easy to understand how the sample was selected and to verify that the sample is representative of the population.
How to Perform Simple Random Sampling?
The following procedures are generally performed in order to do basic random sampling:
- Define the Population: Clearly defining the population of interest is the first stage. This could include any group being studied, as well as individuals, things, and data points.
- Make a Sampling Frame: A sampling frame is a database or list that includes every component of the population. It forms the cornerstone of the procedure for random selection.
- Assign a Unique identity: To make sure that no element is overlooked or tallied more than once, each element in the sampling frame should have a unique identity, such as a serial number.
- Establish the Sample Size: The number of components that the researchers wish to include in the sample must be decided. The correctness of the conclusion drawn from the sample depends critically on the sample size.
- Random Selection: To choose the necessary number of elements from the sample frame, use a random technique, such as drawing lots, using a random number generator, or using a table of random numbers. The procedure ought to be entirely impartial and arbitrary.
- Data Collection: After the sample has been chosen, information is gathered from the selected components. For statistical analysis and inference, these data will be used.
Application of Simple Random Sampling
- Market research: Randomly select a sample of customers to survey about their preferences.
- Quality control: Randomly select a sample of products from an assembly line to inspect for defects.
- Medical research: Randomly assign participants in a study to either receive a new drug or a placebo to compare the outcomes.
- Educational research: Randomly assign students to different classrooms, each of which uses a different teaching method or curriculum, to compare performance.
- Environmental research: Randomly select a sample of locations in a city to measure the levels of air pollution at those locations to create a map of air pollution levels.
When to use Simple Random Sampling?
One simple yet efficient sampling technique that can be applied to a variety of research situations is simple random sampling, or SRS. When the following prerequisites and circumstances are satisfied, it is appropriate:
- Population is Homogeneous: When the population is generally homogeneous—that is, when most of the persons or items within it share a lot of characteristics—simple random sampling performs best. In cases where notable subgroups possess unique attributes, alternative sampling techniques such as stratified sampling may be more suitable.
- Complete Sampling Frame: The sampling frame, which is a list or representation of the complete population, needs to be current and comprehensive. To guarantee that every member of the population has an equal chance of being chosen, the sampling frame is essential.
- Sampling Independence: SRS makes the assumption that each item in the sample was chosen independently of the others. Stated differently, the likelihood of one item in the sample not being included does not change if another item is included. Alternative methods of sampling, such as cluster or systematic sampling, may be more appropriate if dependencies exist.
- Practicality: When money is tight, there are time limits, or other more sophisticated sampling techniques are not practical, simple random sampling is a practical solution because it is reasonably simple to execute.
- Desire for Unbiased outcomes: SRS is a fantastic option if you want to reduce sample bias and strive for unbiased outcomes. By guaranteeing that every individual in the population has an equal chance of being chosen, it lowers risk of systematic errors.
Simple Random vs other sampling methods
selecting people or things at random with equal probability from the population.
|
Homogeneous populations, unbiased results
|
It is easy to implement ,Unbiased
|
May not helpful in heterogeneous population
|
Applying SRS within each stratum after dividing the population into subgroups.
|
Heterogeneous populations with distinct subgroups
|
Can be represented better, More precise estimates for subgroups
|
More Complex
|
selecting a random starting point and then each “kth” element.
|
Quick and simple sampling
|
Can be more efficient than SRS, simplicity
|
Can be biased if there is a pattern
|
dividing the population up into clusters, then choosing a small number of those groups at random for sampling.
|
Geographically dispersed or inaccessible populations
|
cost effective for large population
|
shows low precision, Have Increased variability within clusters
|
Choosing the most practical and reachable people or objects
|
Quick, informal surveys, not for rigorous research
|
low cost , fast and easy to implement
|
Poor representation, High chances of being biased
|
Simple Random Sampling Implementations
Example 1 : Simple Random Sampling from a Tuple
Here are the steps to perform the above random sampling
- Import Libraries
- Define the Population Sample – The population is defined in this phase as a tuple of items. The population in the example is made up of the letters “G,” “E,” “K,” “S,” “F,” “O,” and “R”.
- Determine the Size of the Sample – The sample size, or the number of objects we wish to choose from the population, is specified here.
- Use a Simple random sampling technique – This is the important part when we actually apply a basic random sampling technique. The random module’s ” random.sample() ” function is what we employ.
Python3
import random
population = ( "G" , "E" , "K" , "S" , "F" , "O" , "R" )
sample_size = 4
sample = random.sample(population, sample_size)
print ( "Random Sample:" , sample)
|
Output:
Random Sample: ['S', 'R', 'K', 'O']
Example 2 :Performing Random Sampling on a Titanic dataset
Python3
import random
import pandas as pd
dataset = pd.read_csv(url)
sample_size = 100
random_sample_indices = random.sample( list (dataset.index.values), sample_size)
sample_data = dataset.loc[random_sample_indices]
print (sample_data)
|
Output:
Survived Pclass Name Sex Age \
832 0 3 Mr. Jakob Pasic male 21.0
375 0 1 Mr. Harry Elkins Widener male 27.0
320 1 2 Miss. Hilda Mary Slayter female 30.0
85 0 3 Mr. William Neal Ford male 16.0
457 1 1 Mr. Harry Anderson male 48.0
.. ... ... ... ... ...
34 0 1 Mr. Edgar Joseph Meyer male 28.0
746 1 2 Miss. Joan Wells female 4.0
239 0 3 Miss. Thamine Zabour female 19.0
881 0 3 Mrs. William (Margaret Norton) Rice female 39.0
360 0 3 Mrs. (Catherine David) Barbara female 45.0
Siblings/Spouses Aboard Parents/Children Aboard Fare
832 0 0 8.6625
375 0 2 211.5000
320 0 0 12.3500
85 1 3 34.3750
457 0 0 26.5500
.. ... ... ...
34 1 0 82.1708
746 1 1 23.0000
239 1 0 14.4542
881 0 5 29.1250
360 0 1 14.4542
[100 rows x 8 columns]
Explanation
- The required libraries are imported. To conduct random sampling, we import the random library. To work with data in the DataFrame format, we load pandas.
- We fill a pandas DataFrame with the Titanic dataset. The data is read from the CSV file “train.csv” and stored in the dataset DataFrame via the pd.read_csv() function.
- We choose the desired sample size to be taken from the dataset. The sample size in this example is set to 100, but you can change it to suit your needs.
- We use simple random sampling. To choose random indices from the dataset’s index, we utilize the random.sample() function from the random package. Using list(dataset.index.values), we turn the index into a list to make sure the random.sample() function can handle it as a sequence-like object.
Advantages of Simple Random Sampling
- Unbiased Representation: The application of simple random sampling guarantees that each component of the population has a same probability of being selected for the sample. By doing this, the sample’s chance of bias is reduced.
- Generalizability: The sample is more likely to be representative of the total population because it was chosen at random. This makes it possible for researchers to draw reliable conclusions about the population as a whole.
- Implementation Simplicity: Researchers with varying degrees of experience can easily employ simple random sampling due to its ease of use.
- Transparency: The sampling process’s fairness and unpredictability are obvious and simple to explain to others, which contributes to its widespread acceptance and comprehension.
Disadvantages of Simple Random Sampling:
- Sampling Frame Bias: A biased sample may result from an incomplete or inaccurate sampling frame. A sample that is not representative of the population may result from the exclusion of some individuals.
- Resource-intensive: Establishing a thorough sampling frame and choosing a genuinely random sample can be time- and resource-consuming when dealing with big populations.
- Small Sample Sizes: The precision of the estimates derived from the sample may be limited by simple random sampling, which may lead to smaller sample sizes.
- Practical Difficulties: In certain circumstances, such as when there is nonresponse or when particular elements are hard to obtain, attaining real randomization may be problematic.
Conclusion
A basic statistical technique known as simple random sampling enables researchers to select representative samples from populations for study and inference. Simple random sampling guarantees that the sample accurately reflects the characteristics of the full population by upholding the concepts of equal probability, independence, and real randomness. Despite its drawbacks, this approach is nonetheless a valuable tool for researchers who want to make sense of data gathered from huge and diverse populations. For everyone involved in research or statistics, it is essential to comprehend and apply simple random sampling effectively.
Frequently Asked Questions (FAQs)
1. What is simple random sampling?
Simple random sampling is a statistical method in which every member of a population has an equal chance of being selected into a sample. It is the most basic type of probability sampling.
2. When should I use simple random sampling?
Simple random sampling is best used when you need an unbiased sample of a population and you have a complete and accurate sampling frame. It is also a good choice when the population is small and relatively homogeneous.
3. How do I calculate the sample size for simple random sampling?
The sample size for simple random sampling depends on a number of factors, including the desired margin of error, the confidence level, and the population standard deviation. There are a number of online calculators that can be used to calculate the sample size for simple random sampling.
4. What are some methods of random selection?
Some common methods of random selection include:
- Drawing names out of a hat
- Using a random number generator
- Using a computer program to generate random numbers
5. What is the difference between simple random sampling and stratified random sampling?
Stratified random sampling is a type of probability sampling in which the population is divided into strata, or groups, and then a simple random sample is taken from each stratum. Stratified random sampling is often used to ensure that the sample is representative of the population in terms of important characteristics, such as age, gender, or race.
Share your thoughts in the comments
Please Login to comment...